The challenge is over, but we are running a follow up challenge: the CHALEARN Fast Causation Coefficient Challenge (until June 15, 2014).
Synopsis
The problem of attributing causes to effects is pervasive in science, medicine, economy and almost every aspects of our everyday life involving human reasoning and decision making. What affects your health? the economy? climate changes? The gold standard to establish causal relationships is to perform randomized controlled experiments. However, experiments are costly while non-experimental "observational" data collected routinely around the world are readily available. Unraveling potential cause-effect relationships from such observational data could save a lot of time and effort.
Consider for instance a target variable B, like occurence of "lung cancer" in patients. The goal would be to find whether a factor A, like "smoking", might cause B. The objective of the challenge is to rank pairs of variables {A, B} to prioritize experimental verifications of the conjecture that A causes B.
As is known, "correlation does not mean causation". More generally, observing a statistical dependency between A and B does not imply that A causes B or that B causes A; A and B could be consequences of a common cause. But, is it possible to determine from the joint observation of samples of two variables A and B that A should be a cause of B? There are new algorithms that have appeared in the literature in the past few years that tackle this problem. This challenge is an opportunity to evaluate them and propose new techniques to improve on them.
We provide hundreds of pairs of real variables with known causal relationships from domains as diverse as chemistry, climatology, ecology, economy, engineering, epidemiology, genomics, medicine, physics. and sociology. Those are intermixed with controls (pairs of independent variables and pairs of variables that are dependent but not causally related) and semi-artificial cause-effect pairs (real variables mixed in various ways to produce a given outcome).
This challenge is limited to pairs of variables deprived of their context. Thus constraint-based methods relying on conditional independence tests and/or graphical models are not applicable. The goal is to push the state-of-the art in complementary methods, which can eventually disambiguate Markov equivalence classes. If you are skeptical that this is possible, try this quiz: Examine the plot below of values of variable B plotted as a function of values of variable A. Can you guess which one is a cause of the other? Hint: Some non-linear functions are non-invertible.
A->B or A<-B ?
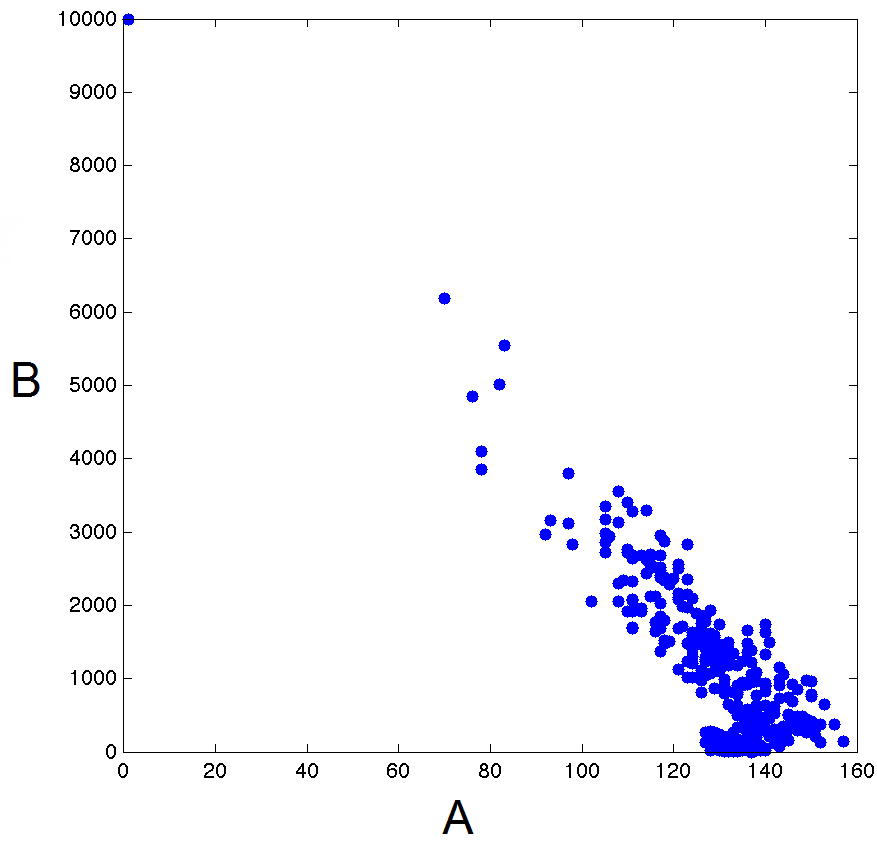
Challenge Rules
- Announcements: To receive announcements and be informed of any change in rules, the participants should subscribe to the Google group causalitychallenge .
- Conditions of participation: Participation requires complying with the rules of the challenge. Prize eligibility is restricted by US government export regulations: any participant from a country under economic sanctions is inelligible for prizes (see the OFAC website for an up-to-date list); the restrictions also extends to designated individuals. Are also excluded from prize eligibility:
- The Competition organizers and sponsors, their students, close family members (parents, sibling, spouse or children) and household members.
- In track 2 (quantitative evaluation), any person having had access to the truth values or to any information about the data or the challenge design giving him (or her) an unfair advantage.
- The CHALEARN employees, Directors, Officers, and Advisors, and their students, close family members (parents, sibling, spouse or children) and household members.
- The member of the expert committee appointed to judge the challenge and/or papers.
A disqualified person may submit one or several entries in the challenge and request to have them evaluated, provided that they notify the organizers of their conflict of interest. If a disqualified person submits an entry, this entry will not be part of the final ranking and does not qualify for prizes. The participants should be aware that CHALEARN and the organizers reserve the right to evaluate for scientific purposes any entry made in the challenge, whether or not it qualifies for prizes.
- Tracks: There are two participation tracks:
- As a data donor by providing query pairs of variables (with known, unknown or suspected causal relationship).
- As a problem solver by submitting results on provided cause-effect pairs.
A participant may enter both tracks. The cause-effect pairs provided by the donors will be used for benchmarking algorithms but THEY WILL NOT BE USED FOR SCORING THE CHALLENGE.
- Dissemination:
This challenge is part of the official selection of IJCNN 2013, August 4-9, 2013. The top ranking participants will also be invited to present at a NIPS 2013 conference workshop on causality in December 2013 (pending acceptance). To present at the NIPS workshop, abstracts must be submitted before the deadline (see the updated schedule) to causality@chalearn.org. Participants are not required to attend these events to qualify for prizes.
The proceedings of the competition will be published by the Journal of Machine Learning Research, Workshop and Conference Proceedings (JMLR).
- Anonymity: The participants who do not submit a paper to the workshop can elect to remain anonymous. Their results will be published, but their name will remain confidential. See our privacy policy.
- Submission method: The results must be submitted to the Kaggle website. The participants can make up to 5 submissions per day and select
up to 3 final submissions ONLY ONE FINAL SUBMISSION (final rule). The participants must adhere to the rules edicted by Kaggle to make entries. In case of problem, send email to causality@chalearn.org.
- Evaluation and rewards: To compete towards the prizes, the participants must submit a 6-page paper describing their donated dataset and the findings derived from the challenge results (if they entered as a donor, track 1), or their task-solving method(s) and result(s) (if they entered as a solver, track 2), before the submission deadline (see the updated schedule) to causality@chalearn.org (A sample paper and a Latex style file are provided). The challenge participants must append their fact sheet to their paper, see template provided in Latex. Each participant is allowed to submit two papers, on in each tracks. The papers will be peer reviewed and several prizes will be awarded (see details). Only the entries of accepted papers will qualify for prizes.
- Reproducibility: To qualify for prizes, the participants must submit their software prior to the deadline (see the updated schedule) and cooperate with the organizers to reproduce their entries. This will include filling out a fact sheet about their methods. The winners will be required to make their code publicly available under a popular OSI-approved license, if they accept their prize, within a week of the deadline for submitting the final results.
Schedule (updated June 28, 2013)
- Thursday, March 28, 2013: Challenge start.
- Friday, May 17: Track 1: Deadline for submission of cause-effect pairs for benchmark purposes (but not counting towards scoring in track 2).
- Monday, May 20, 2013: Track 2: Release of supplementary data.
- Monday, July 1, 2013: Track 2: Release of final training and validation data and encrypted test data.
- Monday,
July 15 August 19, 2013: Track 2: Deadline for submitting software.
- Friday,
July 19 August 23, 2013: Track 2: Release of test data decryption key.
- Friday,
July 26 August 30, 2013 September 2, 2013: Track 2: Deadline for submitting results. End of challenge.
- Friday,
August 2 September 6, 2013: Track 2: Deadline for the winners to make their code publicly available.
-
Monday, September 16 Wednesday October 9, 2013: JMLR proceedings paper and abstract submission deadline.
-
Tuesday, October 15, Wednesday October 23, 2013: Paper notification of acceptance.
- December 9 or 10, 2013: NIPS 2013, Tahoe, Nevada, USA. Workshop accepted.